AI Helped Me: Creating a tool for quick marketing briefs in a regulated industry
The tool can automate repetitive tasks so humans can do the fun parts.
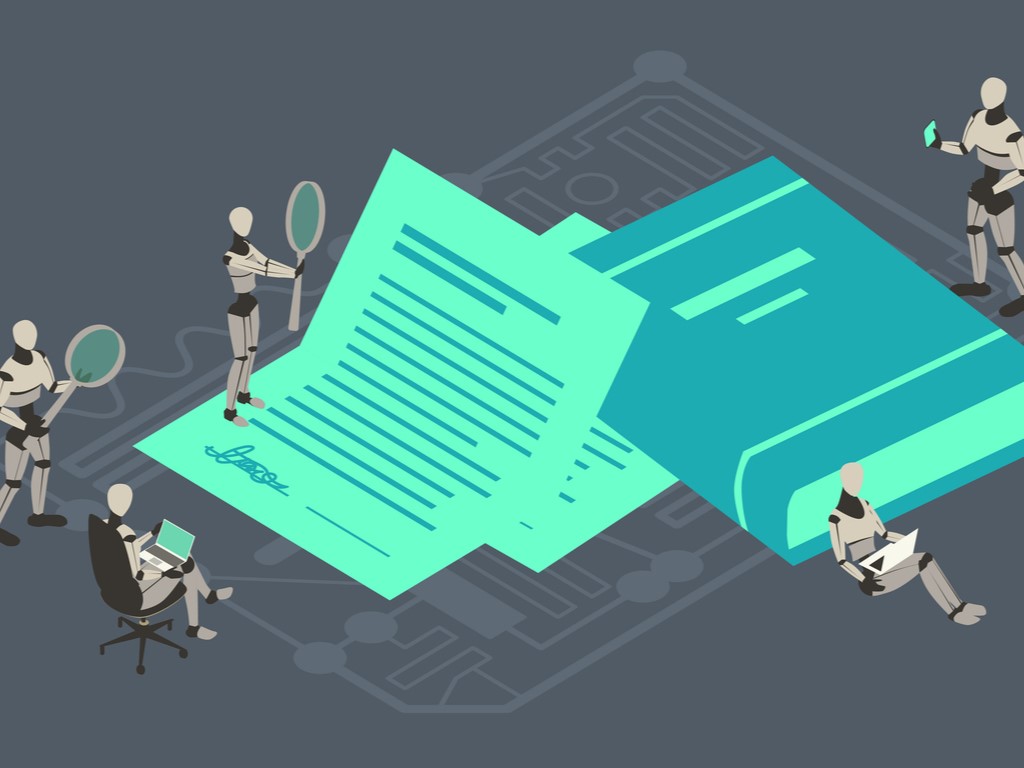
1474442258
In marketing, there are so many activities that are tedious. Whether that’s updating dozens of banner ads to reflect new legal disclosures or writing a marketing brief, AI is increasingly a way to reduce the tedium and increase creativity in any marketer’s day.
Chris Cullmann, chief innovation officer at RevHealth, and Douglas Barr, AI lead and founder at PixieDust Labs, worked together to create a tool that would cut down on some of these headaches while also understanding the unique regulatory challenges in healthcare. So AgencyOS was born.
Here’s how the idea came together – and here’s what’s next.
Answers have been edited for brevity and clarity.
How did you come up with the idea for an AI that could give a marketing brief in seven minutes?
Cullmann: In conversations with Doug, we were forecasting the role artificial intelligence is going to play into not just the search market, but the experiential market for any industry where access to accurate information is important. In our exploring ways to build out structures around accuracy, removing hallucinations and limiting the data sets a manufacturer wants access to the most relevant information about their therapies in the context of treating patients, we started talking, very casually about, what if you know we could do this? What are the challenges inside of an agency that we could leverage these same technologies for? And from that, Doug built a prototype that was fascinatingly close to the iteration that that launched us into the solution that you’re talking about.
Barr: When we started thinking about our understanding of healthcare, healthcare data and content generation, there was a perfect lead-in to generating quality, solving some of the more complex problems within healthcare and content generation.
Obviously regulatory needs were top of mind. Data privacy, I’m sure, was a huge issue. Walk me through how you addressed some of those issues.
Barr: We put safeguards into place and privacy protection, more on the code side of things, that allowed us to maintain privacy and make sure that we were compliant with all the privacy acts and HIPAA. So that was actually a very complex problem to solve … So we had to develop a couple of intellectual property models to help prevent the hallucinations from occurring and make sure it didn’t divulge any private data, things that weren’t into market. We began with using in-market, publicly available content, and Chris and the partners there allowed us to consume that type of knowledge, which lessened the restrictions on what we had to what we were getting out of it, and to make sure that again, it wasn’t out of compliancy. So that was the beginning of how we began there.
Cullmann: I also think the partnership between RevHealth and Pixie Dust allowed us to be able to explore models that worked with incremental steps to a potentially automated future, but more importantly, one where we’re allowing our team members to completely interact and inform that model.
This allows our team members, when they’re working with the platform, to review and verify the information that’s coming out of the artificial intelligence, and one that allows us to be able to have our teams augmenting this. The brief process itself, timelines for all the individual projects that go through, especially in a regulated industry like ours, all of them need due diligence. It’s incredibly repetitive, and for organizations like ours that thrive on creativity and strategy and the human spark combating the fatigue of that day-to-day work with the achievement and the creative process is one that needs to be balanced. AgencyOS allows our team members to really focus in on the creative process, strategy process and being able to interact with our clients to be able to refine what the best solution is for them. From a communications standpoint, by removing a lot of the repetitive actions, we really are creating an opportunity for much fertile exchange of ideas and to challenge those more complex jobs, to have more time and for us to explore more challenging ideas.
Barr: The other aspect of it that’s remarkable is the fact that we’re able to take that human feedback and adjustment and through what’s called a reinforcement learning, or an RL, algorithm. That feedback goes back into the model as part of the human in the loop.
So tell me, what is the output of AgencyOS like today? You put in your parameters, you get out a brief, what does that look like? How much time do you have to spend editing and refining it? What does that process look like?
Cullmann: When the internet was much, much earlier on, I think there was a lot of nuance around search. The quality of the search returns you got were very much related to how we were able to put in search. The same thing is true when we begin working with prompts. Doug’s team has built out a process inside of agency OS that automatically refines some of the prompts through an engineering process.
It will prompt you for additional information if you leave out deadlines or requirements. It understands some of the nuances of medium. It understands what an email is, what a banner ad is. It understands the requirements of Facebook, X, TikTok from a structure standpoint. It understands what marketing objectives look like. It also understands, when trained against a specific clinical claims library, those libraries of the disease state that allow a client’s product to have a unique value in the marketplace, and all of the justification for an FDA approval that’s associated with that. All of the due diligence is folded into that claims library, which means, when trained, it can not only create emails, but also be able to create emails that are pertinent to our clients’ unique value proposition in the marketplace and its unique value proposition to a physician as to how they might choose that for a specific patient, assuming the patient meets the profile.
Barr: One of the differentiators between our platform and something like ChatGPT, where ChatGPT is a single agent. What we what we’ve done with our platforms is that we have multiple versions. Each represents a separate role within an organization. We have a senior project manager, we have a creative director, we have just a straight-up project manager, we have a strategist involved, and we have a programmer. And then we can scale these. They all communicate with each other to accomplish a specific task.
Cullmann: A product may change the indication, the solution or the patient that would be approved for the FDA for it, … we could quickly iterate on many different changes that could be through those tactics, thus changing the speed of response we can have in making this more compliant as an industry. When you’re a person and you spent the last year working on a specific indication, it’s very hard to pivot when you’re doing a lot of these repetitive tasks. If you had to update 20 or 30 banner ads with the label information, the likelihood of a mistake dramatically increases as you go through those repetitive tasks.
So what’s next? Now that you’ve got this tool, how are you going to keep building on it? And how do you see AI helping in that?
Barr: At Pixie Dust, we actually have two challenges that we’re looking to solve in the future. The first is more immediate. We’ve demonstrated a platform to people, and the truth be told, some of them have pulled me aside and said, “the team’s terrified.” So we have to educate people to understand what it does correctly, what it doesn’t do correctly, how people are still involved and need to be involved. We actually have to spend a lot more resources into educating people on the technology, which is kind of surprising to us. We thought, we developed this, and everyone’s gonna jump on board and use it, but there’s that fear that’s involved in, what does that do to our business model?
And the second thing was, we want to focus on what are called vision models. So currently, large language models are exactly that. They’re language models. It essentially predicts the next word and it writes it out. But that’s only one half of the world. The other half of the world is vision based. It’s through video, or it’s through images. So vision models are models that allow us not just that creative output, like Adobe Firefly, where it generates images. What I’m really describing is how these models see and interpret the visual world around them. For example, you can upload an image of a graph and start asking the model questions about the data points in the graph from an image. We can do that work today. That world needs to be expanded upon to make better use of it.
Cullmann: As businesses begin to use this, I think there’s a lot of initial fears: we can’t put our proprietary data into the cloud for general collection. So there needs to be a lot more nuanced understanding as to data and data rules as to, this is my company’s data. This is my client’s data. This is public data, and managing that and what platform you choose to manage that are all important elements to the decision making process as to how you’re using this to the risk and reward benefit.